Tutorial I
Domenico Spina,Vrije Universiteit Brussel
Tom DHaene,Ghent Unviersity
Abstract -
In recent years, machine learning has emerged as an essential tool in the design and analysis of complex RF and microwave systems. This tutorial aims to familiarize the audience with the innovative contributions of machine learning in this field and highlight the latest advancements. The tutorial will start with a discussion of the motivation for the adoption of machine learning in RF and microwave design, and demonstrate how it addresses key challenges in the sector. A description of the fundamentals of machine learning will follow, and suitable applications to RF and microwave design problems will be presented. The tutorial will cover both Neural Networks-based and data-efficient Bayesian methodologies, illustrating their properties and discussing their capability to solve different design problems. Relevant microwave examples will illustrate the application of the proposed machine learning methodologies.
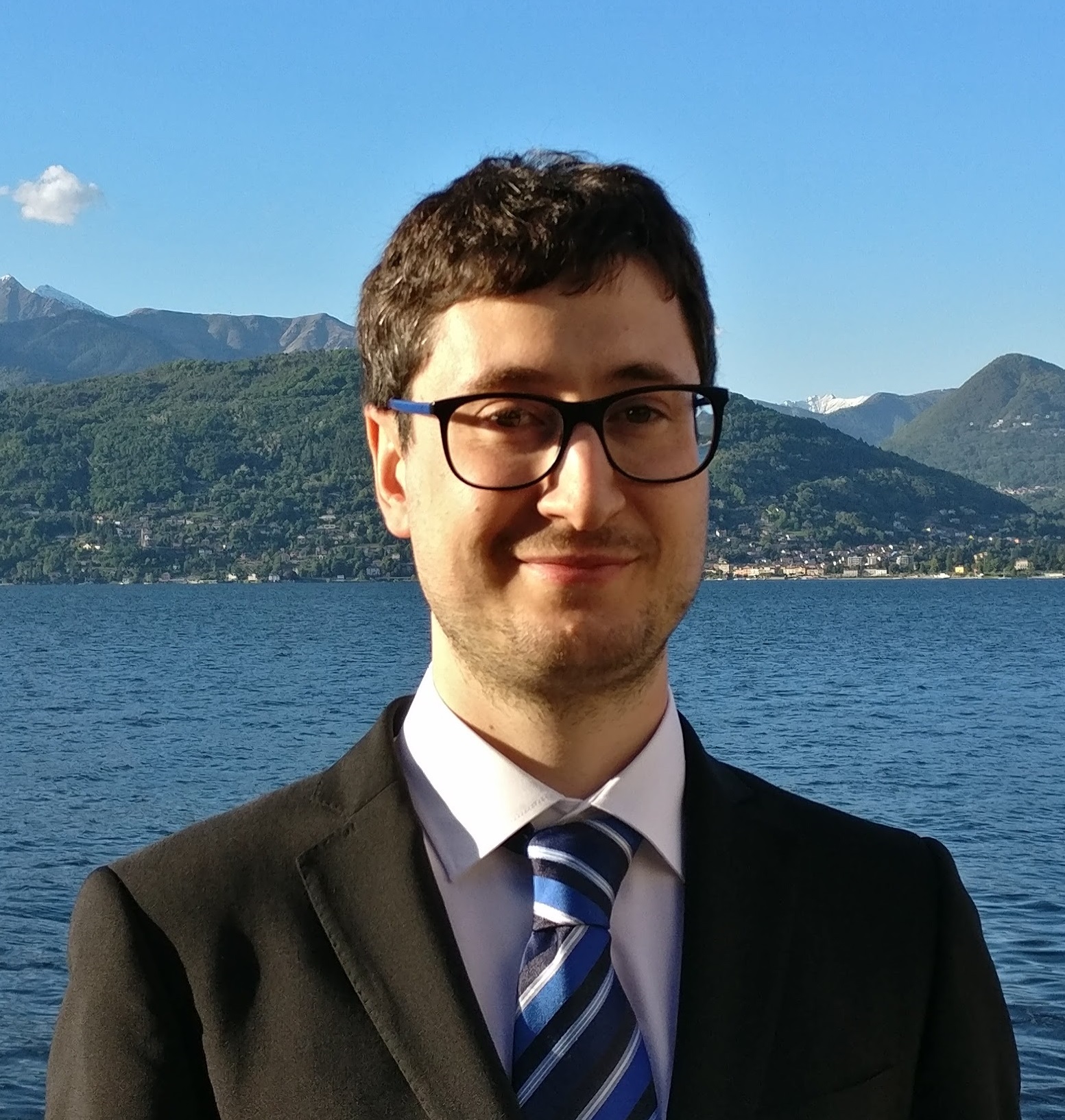
Domenico Spina (Senior Member, IEEE) received the M.S. degree (cum laude) in electronics engineering from the University of L'Aquila in 2010, and a joint Ph.D. degree in electrical engineering from the Ghent University and the University of L'Aquila in 2014. From 2015 until 2023, he was a member of the Internet and Data Science Lab (IDLab) of the Department of Information Technology (INTEC) at Ghent University - IMEC, first as postdoctoral researcher and then as R&D technical lead. He is currently a professor at the ELEC department of the Vrije Universiteit Brussel, where he is establishing a research group in the field of physics-inspired AI for analog electronic circuits. His current research interests include machine learning based design of high frequency analog circuits, system identification, and uncertainty quantification.

Tom Dhaene is a Full Professor at Ghent University in Belgium and is affiliated with IMEC, a leading R&D and innovation center in nanoelectronics and digital technologies. He teaches a course on Machine Learning for master's students in Engineering. As an author or co-author, Tom Dhaene has contributed to over 500 peer-reviewed papers and abstracts. His modeling and simulation software is utilized worldwide by academic, governmental, and business organizations for the study, design, and optimization of high-speed electronics and other complex engineering systems. His current research interests include surrogate modeling, data-efficient machine learning (DEML), physics-inspired AI, and Bayesian optimization.